From Neural Networks to Reinforcement Learning to Game Theory
Academics and industry experts shared their latest research and the broader potential of AI during The New York Academy of Sciences’ 2025 Machine Learning Symposium.
Published November 14, 2024
By Nick Fetty
Digital Content Manager
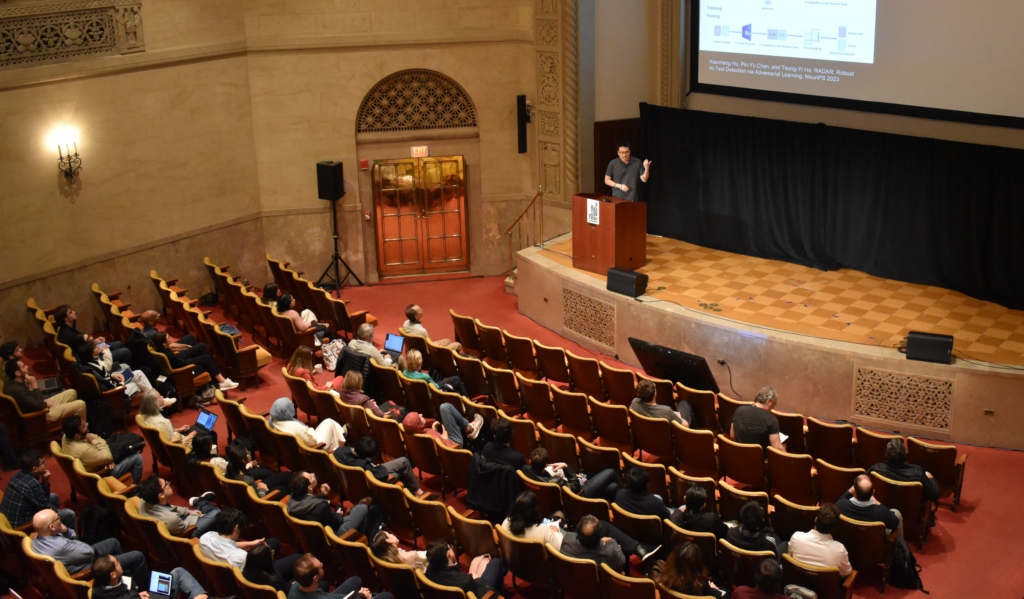
The New York Academy of Sciences (the Academy) hosted the 15th Annual Machine Learning Symposium at the New York Academy of Medicine on October 18, 2024. This year’s event, sponsored by Google Research and Cubist Systematic Strategies, included keynote addresses from leading experts, spotlight talks from graduate students and tech entrepreneurs, and opportunities for networking.
Exploring and Mitigating Safety Risks in Large Language Models and Generative AI
Pin-Yu Chen, PhD, a principal research scientist at IBM Research, opened the symposium with a keynote lecture about his work examining adversarial machine learning of neural networks for robustness and safety.
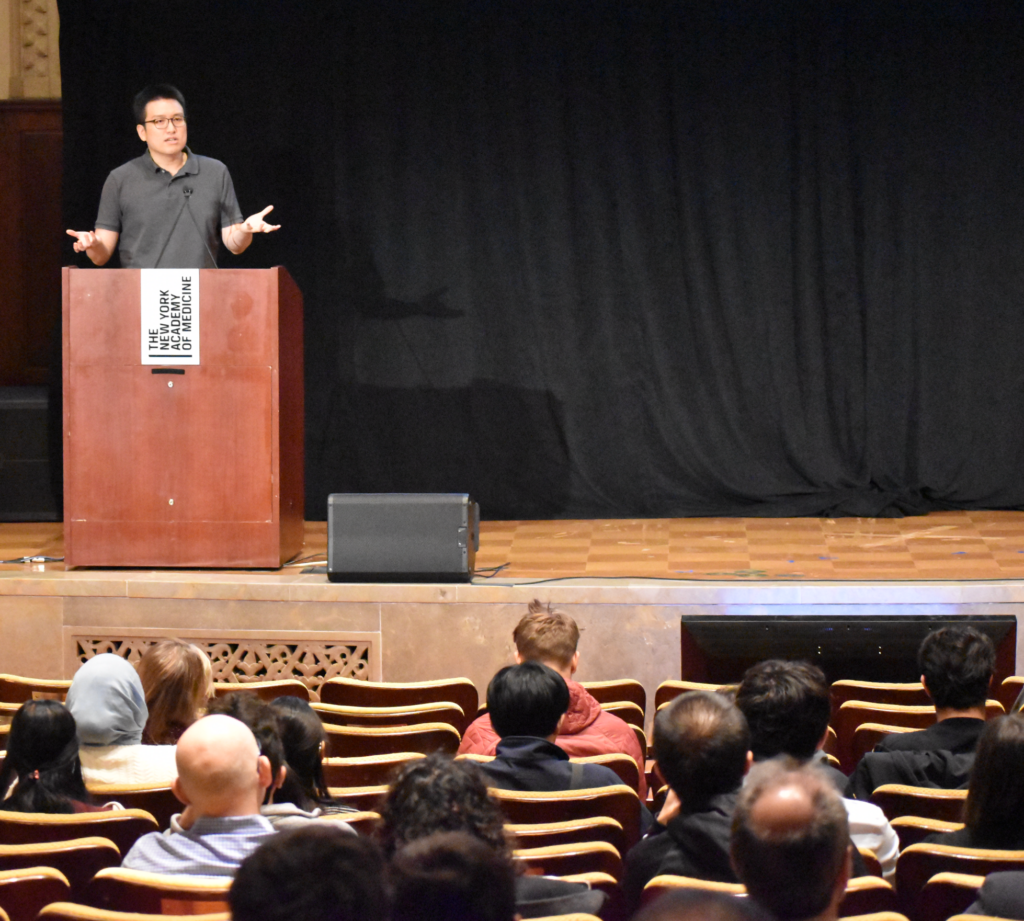
Dr. Chen presented the limitations and safety challenges facing researchers in the realm of foundation models and generative AI. Foundation models “mark a new era of machine learning,” according to Dr. Chen. Data sources, such as text, images, and speech, help to train these foundation models. These foundation models are then adapted to perform tasks ranging from answering questions to object recognition. ChatGPT is an example of a foundation model.
“The good thing about foundation models is now you don’t have to worry about what task you want to solve,” said Dr. Chen. “You can spend more effort and resources to train a universal foundation model and fine-tune the variety of the downstream tasks that you want to solve.”
While a foundation model can be viewed as an “one for all” solution, according to Dr. Chen, generative AI is on the other side of the spectrum and takes an “all for more” approach. Once a generative AI model is effectively trained with a diverse and representative dataset, it can be expected to generate reliable outputs. Text-to-image and text-to-video platforms are two examples of this.
Dr. Chen’s talk also brought in examples of government action taken in the United States and in European Union countries to regulate AI. He also discussed “hallucinations” and other bugs occurring with current AI systems, and how these issues can be further studied.
“Lots of people talk about AGI as artificial general intelligence. My view is hopefully one day AGI will mean artificial good intelligence,” Dr. Chen said in closing.
Morning Short Talks
The morning session also included a series of five-minute talks delivered by early career scientists:
- CoLoR-Filter: Conditional Loss Reduction Filtering for Targeted Language Model Pre-training
David Brandfonbrener, PhD, Harvard University - On the Benefits of Rank in Attention Layers
Noah Amsel, BS, Courant Institute of Mathematical Sciences - A Distributed Computing Lens on Transformers and State-Space Models
Clayton Sanford, PhD, Google Research - Efficient Stagewise Pretraining via Progressive Subnetworks
Abhishek Panigrahi, Bachelor of Technology, Princeton University - MaxMin-RLHF: Towards Equitable Alignment of Large Language Models with Diverse Human Preferences
Souradip Chakraborty, PhD, University of Maryland
Revisiting the Exploration-Exploitation Tradeoff in the Era of Generative Sequence Modeling
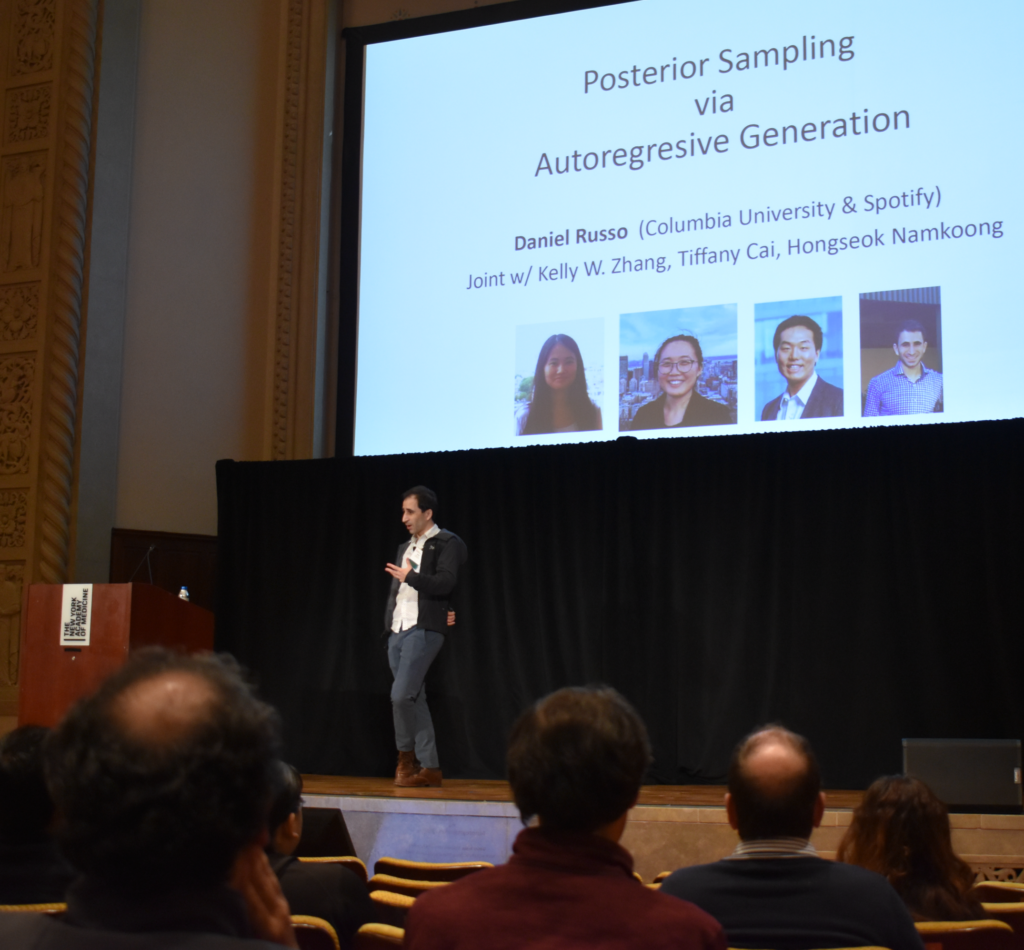
Daniel Russo, PhD, the Philip H. Geier Jr. Associate Professor of Business at Columbia University, delivered a keynote about reinforcement learning. This field combines statistical machine learning with online decision-making. Prof. Russo covered the work that has taken place in his lab over the past year.
He pointed out that today, “humans have deep and recurring interactions with digital services that are powered through versions of AI.” This includes everything from platforms for dating and freelance work, to entertainment like Spotify and social media, to highly utilitarian applications such as for healthcare and education.
“The thing I deeply believe is that decision making among humans involves information gathering,” said Prof. Russo. “It involves understanding what you don’t know about the world and figuring out how to resolve it.”
He said medical doctors follow a similar process as they assess what might be affecting a patient, then they decide what tests are needed to better diagnose the issue. MDs must weigh the costs versus the benefits. Prof. Russo pointed out that in their current state, it’s difficult to design machine learning agents to effectively make these assessments.
He then discussed major advancements in the field that have occurred over the past decade and did a deep dive into his work on generative modeling. Prof. Russo closed his talk by emphasizing the difficulty of quantifying uncertainty in neural networks, despite his desire to be able to program them for decision-making.
“I think what this [research] is, is the start of something. Definitely not the end,” he said. “I think there’s a lot of interesting ideas here, so I hope that in the years to come this all bears out.”
Award-Winning Research
Researchers, ranging from high schoolers to industry professionals, shared their projects and work with colleagues during the popular poster session. Graduate students, postdocs, and industry professionals delivered a series of spotlight talks. Conference organizers assessed the work and presented awards to the most outstanding researchers. Awardees include:
Posters:
- Aleksandrs Slivkins, PhD, Microsoft Research NYC (his student, Kiarash Banihashem, presented on his behalf)
- Aditya Somasundaram, Bachelor of Technology, Columbia University
- R. Teal Witter, BA, New York University
Spotlight Talks:
- Noah Amsel, BS, Courant Institute of Mathematical Sciences
- Claudio Gentile, PhD, Google
- Anqi Mao, PhD, Courant Institute of Mathematical Sciences
- Tamalika Mukherjee, PhD, Columbia University
- Clayton Sanford, PhD, Google Research
- Yutao Zhong, PhD, Courant Institute of Mathematical Sciences
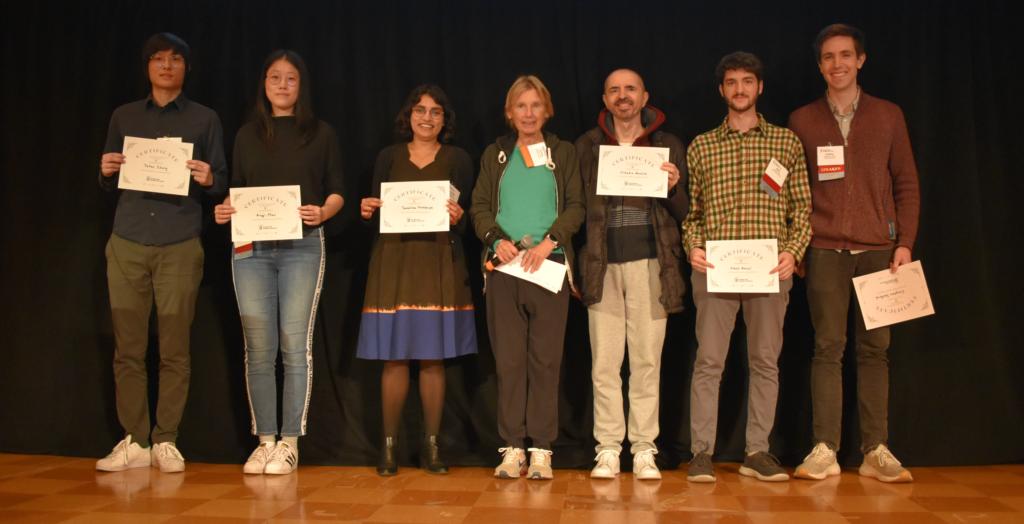
Playing Games with Learning Agents
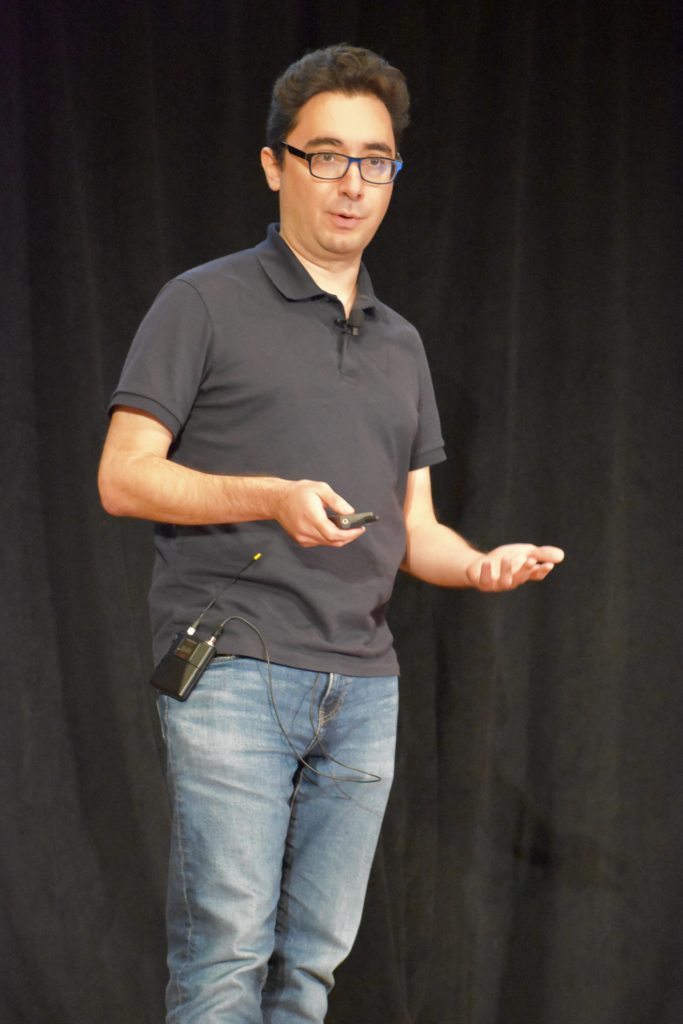
To start the afternoon sessions, Jon Schneider, PhD, from Google Research New York, shared a keynote covering his research at the intersection of game theory and the theory of online learning.
“People increasingly now are offloading their decisions to whatever you want to call it; AI models, learning algorithms, automated agents,” said Dr. Schneider. “So, it’s increasingly important to design good learning algorithms that are capable of making good decisions for us.”
Dr. Schneider’s center of expertise and research involves decision-making in strategic environments for both zero-sum (rock-paper-scissors) and general-sum games (chess, Go, StarCraft). He shared some examples of zero-sum games serving as success stories for the theories of online learning and game theory. In this realm, researchers have observed “tight connections” between the economic theory and the theory of learning, finding practical applications for these theoretical concepts.
“Thinking about these convex objects, these menus of learning algorithms, is a powerful technique for understanding questions in this space. And there’s a lot of open questions about swap regret and the manipulative-ability of learning algorithms that I think are still waiting to be explored,” Dr. Schneider said in closing.
Afternoon Short Talks
Short talks in the afternoon by early career scientists covered a range of topics:
- Improved Bounds for Learning with Label Proportions
Claudio Gentile, PhD, Google - CANDOR: Counterfactual ANnotated DOubly Robust Off-Policy Evaluation
Aishwarya Mandyam, MS, Stanford University - Cardinality-Aware Set Prediction and Top-k Classification
Anqi Mao, PhD, Courant Institute of Mathematical Sciences - Cross-Entropy Loss Functions: Theoretical Analysis and Applications
Yutao Zhong, PhD, Courant Institute of Mathematical Sciences - Differentially Private Clustering in Data Streams
Tamalika Mukherjee, PhD, Columbia University
Towards Generative AI Security – An Interplay of Stress-Testing and Alignment
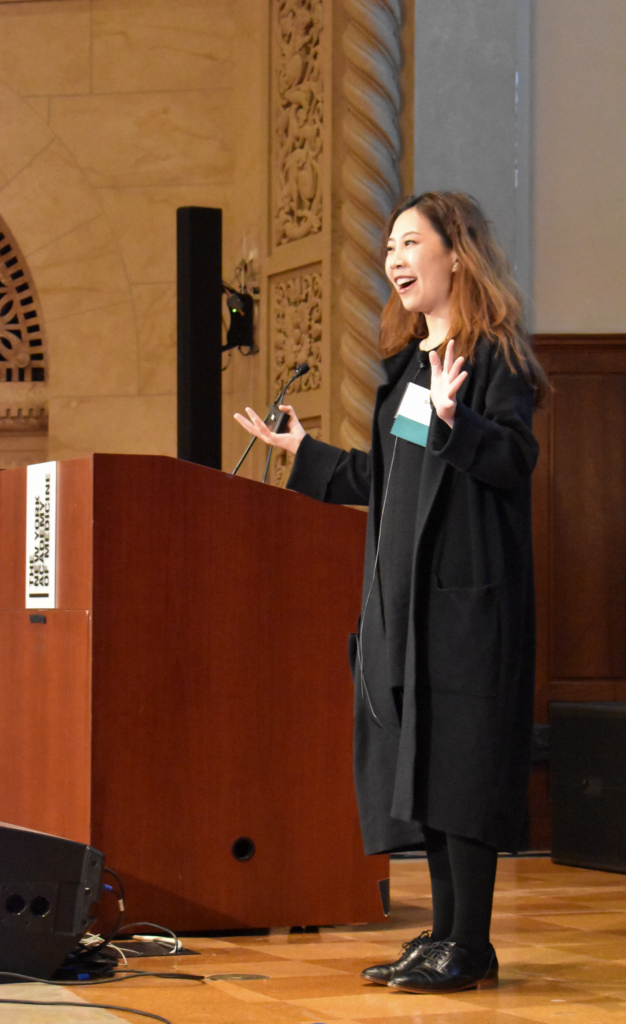
The event concluded with a keynote talk from Furong Huang, PhD, an associate professor of computer science at the University of Maryland. She recalled attending the Academy’s Machine Learning symposium in 2017. She was a postdoctoral researcher for Microsoft Research at the time, and had the opportunity to give a spotlight talk and share a poster. But she said she dreamt of one day giving a keynote presentation at this impactful conference.
“It took me eight years, but now I can say I’m back on the stage as a keynote speaker. Just a little tip for my students,” said Prof. Huang, which was met by applause from those in attendance.
Her talk touched on large language models (LLMs) like ChatGPT. While other popular programs like Spotify and Instagram took 150 days and 75 days, respectively, to gain one million users, ChatGPT was able to achieve this benchmark in just five days. Furthermore, Prof. Huang pointed out the ubiquity of AI in society, citing data from the World Economic Forum, which suggests that 34% of business products are produced using AI, or augmented by AI algorithms.
AI and Public Trust
Despite the ubiquity of the technology (or perhaps because of it), she points out that public trust of AI is lacking. Polling shows a strong desire from Americans to make AI safe and secure. She went on to explain that for public trust to be gained, LLMs and visual language models (VLMs) need to be better calibrated to avoid behavioral hallucinations. This happens when the AI misreads situations and infers behaviors that aren’t actually occurring. Prof. Huang concluded by emphasizing the utility of stress-testing when developing AI systems.
“We use stress-testing to figure out the vulnerabilities, then we want to patch them. So that’s where alignment comes into play. Using the data we got from stress-testing, we can do training time and test time alignment to make sure the model is safe,” Prof. Huang concluded, adding that it may be necessary to conduct another round of stress-testing after a system is realigned to further ensure safety.
Want to watch these talks in their entirety? The video is on-demand for Academy members. Sign up today if you aren’t already part of our impactful network.