A More Scientific Approach to Artificial Intelligence and Machine Learning
Taking a more scientific perspective, while remaining ethical, can improve public trust of these emerging technologies.
Published August 13, 2024
By Nitin Verma, PhD
AI & Society Fellow
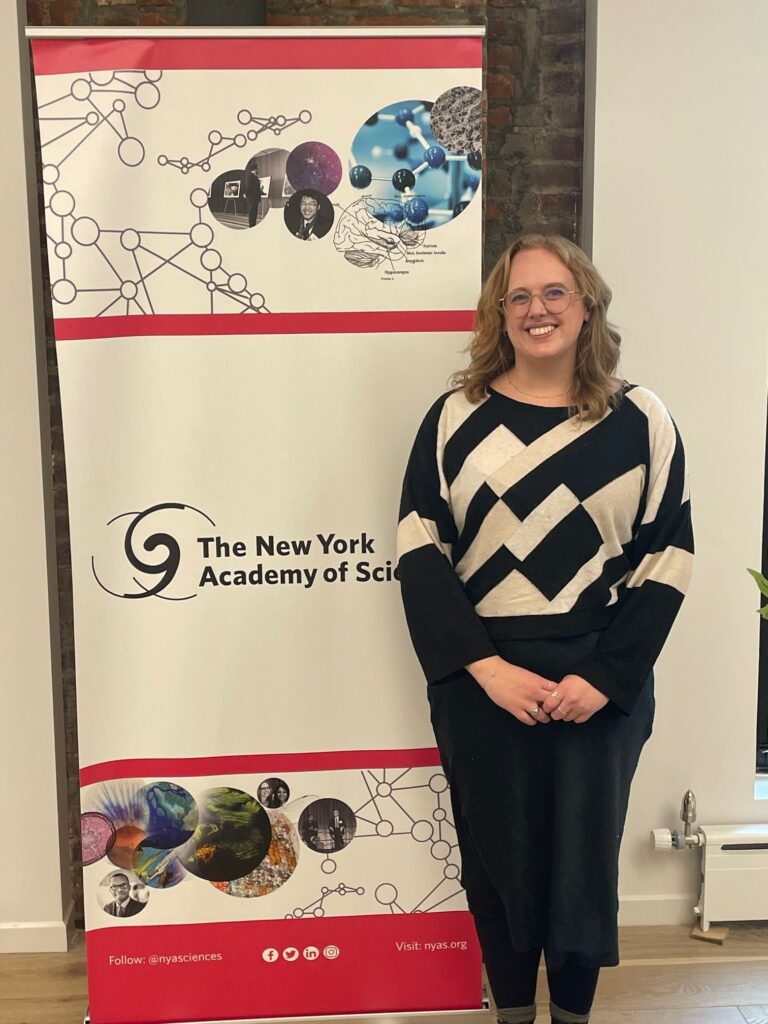
Savannah Thais, PhD, is an Associate Research Scientist in the Data Science Institute at Columbia University with a focus on machine learning. Dr. Thais is interested in complex system modeling and in understanding what types of information is measurable or modelable and what impacts designing and performing measurements have on systems and societies.
*This interview took place at The New York Academy of Sciences on January 18, 2024. This transcript was generated using Otter.ai and was proofread for corrections. Some quotes have been edited for length and clarity*
Tell me about the big takeaways from your talk?
The biggest highlight is that we should be treating machine learning and AI development more scientifically. I think that will help us build more robust, more trustworthy systems, and it will help us better understand the way that these systems impact society. It will contribute to safety, to building public trust, and all the things that we care about with ethical AI.
In what ways can the adoption of scientific methodology make models of complex systems more robust and trustworthy?
I think having a more principled design and evaluation process, such as the scientific method approach to model building, helps us realize more quickly when things are going wrong, and at what step of the process we’re going wrong. It helps us understand more about how the data, our data processing, and our data collection contributes to model outcomes. It helps us understand better how our model design choices contribute to eventual performance, and it also gives us a framework for thinking about model error and a model’s harm on society.
We can then look at those distributions and back-propagate those insights to inform model development and task formulation, and thereby understand where something might have gone wrong, and how we can correct it. So, the scientific approach really just gives us the principles, and a step-by-step understanding of the systems that we’re building. Rather than, what I see a lot of times, a hodgepodge approach where the only goal is model accuracy, in which something goes wrong, we don’t necessarily know why or where.
You have a very interesting background, and your work touches on various academic disciplines, including machine learning, particle physics, social science, and law. How does this multidisciplinary background inform your research on AI?
I think being trained as a physicist really impacts how I think about measurements and system design. We have a very specific idea of truth in physics. And that isn’t necessarily translatable to scenarios where we don’t have the same kind of data or the same kind of measurability. But I think there’s still a lot that can be taken from that, that has really informed how I think about my research in machine learning and its social applications.
This includes things like experimental design, data validation, uncertainty, propagation in models. Really thinking about how we understand the truth of our model, and how accurate it is compared to society. So that kind of idea of precision and truth that’s fundamental physics, has affected the research that I do. But my other interests and other backgrounds are influential as well. I’ve always been interested in policy in particular. Even in grad school, when I was doing a physics PhD, I did a lot of extracurricular work in advocacy in student government at Yale. That impacted a lot how I think about understanding how systems affect society, resource access, and more. It really all mixes together.
And then the other thing that I’ll say here is, I don’t think one person can be an expert in this many things. So, I don’t want it to seem like I’m an expert at law and physics and all this stuff. I really lean a lot on interdisciplinary collaborations, which is particularly encouraged at Columbia. For example, I’ve worked with people at Columbia’s School of International and Public Affairs as well as with people from the law school, from public health, and from the School of Social Work. My background allows me to leverage these interdisciplinary connections and build these truly collaborative teams.
Is there anything else you’d like to add to this conversation?
I would reemphasize that science can help us answer a lot of questions about the accuracy and impact of machine learning models of societal phenomena. But I want to make sure to emphasize at the same time that science is only ever going to get us so far. And I think there’s a lot that we can take from it in terms of experimental design, documentation, principles, model construction, observational science, uncertainty, quantification, and more. But I think it’s equally important that as scientific researchers, which includes machine learning researchers, we really make an effort to both engage with other academic disciplines, but also to engage with our communities.
I think it’s super important to talk to people in your communities about how they think about the role of technology in society, what they actually want technology to do, how they think about these things, and how they understand them. That’s the only way we’re going to build a more responsible, democratic, and participatory technological future. Where technology is actually serving the needs of people and is not just seen as either a scientific exercise or as something that a certain group of people build and then subject the rest of society to, whether it’s what they actually wanted or not.
So I really encourage everyone to do a lot of community engagement, because I think that’s part of being a good citizen in general. And I also encourage everyone to recognize that domain knowledge matters a lot in answering a lot of these thorny questions, and that we can make ourselves better scientists by recognizing that we need to work with other people as well.
Also read: From New Delhi to New York