Studying and Implementing Dimensionality Reduction
The Academy community represents one of the most dynamic and diverse groups of STEM professionals and science enthusiasts and supporters around the world, with more than 16,000 Members across 100 countries.
Published May 1, 2016
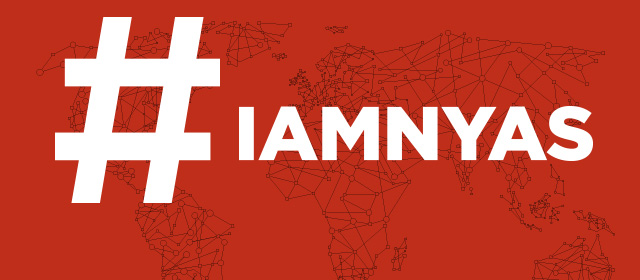
Academy Members are building STEM careers, overcoming the challenges associated with cutting-edge research, putting science into practice, influencing policy, and supporting future generations of science leaders.
We invite you to get to know your fellow Academy Members and learn about new opportunities to interact and get involved!
Dmitry Storcheus
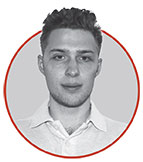
Dmitry Storcheus, MS, is an engineer at Google Research NY, where he specializes in the research and implementation of dimensionality reduction.
What initially drew you to the field of machine learning?
I was drawn to the field because of the remarkable power of machine learning tools to learn and forecast patterns in data. I remember an article from 2011 about scientists from Stanford who were able to use machine learning to study breast cancer with their algorithm (called C-Path) using microscopic images. They reported that the algorithm was more accurate than human doctors in predicting survival, which was amazing for me at that time. The success of machine learning combined with its mathematical rigor inspired me to conduct research in this field.
What are some of the biggest challenges in machine learning right now?
The first one is regarding supervised versus unsupervised methods. While unsupervised methods have greater flexibility, the supervised ones can be fine-tuned to achieve better accuracy, so there is a tradeoff. Recently I published a paper coauthored with Mehryar Mohri and Afshin Rostamizadeh that makes a point for using supervised dimensionality reduction, since it has favorable learning guarantees. Particularly, we show that the generalization error of a hypothesis class that includes learning a linear combination of kernels that define projection jointly with a classifier has a favorable bound.
The second challenge is “Can kernel machines match deep neural networks in accuracy?” So far we have seen great progress by wonderful scientists, such as Fei Sha and Le Song, who were able to use kernel approximations to match deep neural networks in accuracy on speech datasets and provide theoretical justification of their results. This work is still in progress, and I think it will be raising widespread discussions in the next couple of years.
Expand Your Network!
Inspired by the passion, expertise, and unique perspectives of your fellow Members? Tap in to the incredible network the Academy offers through our mentoring programs.
We’re thrilled to offer you access to a new opportunity to get involved and interact: Member-to-Member Mentoring. The program matches you with a mentor—or a mentee—who is a fellow STEM professional and Academy Member. Depending upon your experience level and needs, you can request a mentor, become a mentor, or both.
Interested in mentoring students? We also offer incredible mentorship opportunities through the Academy’s Global STEM Alliance, which delivers education programs that can help you develop your teaching and communication skills, while paying it forward to the next generation of scientific innovators.
Peruse our mentorship opportunities, and sign up today!