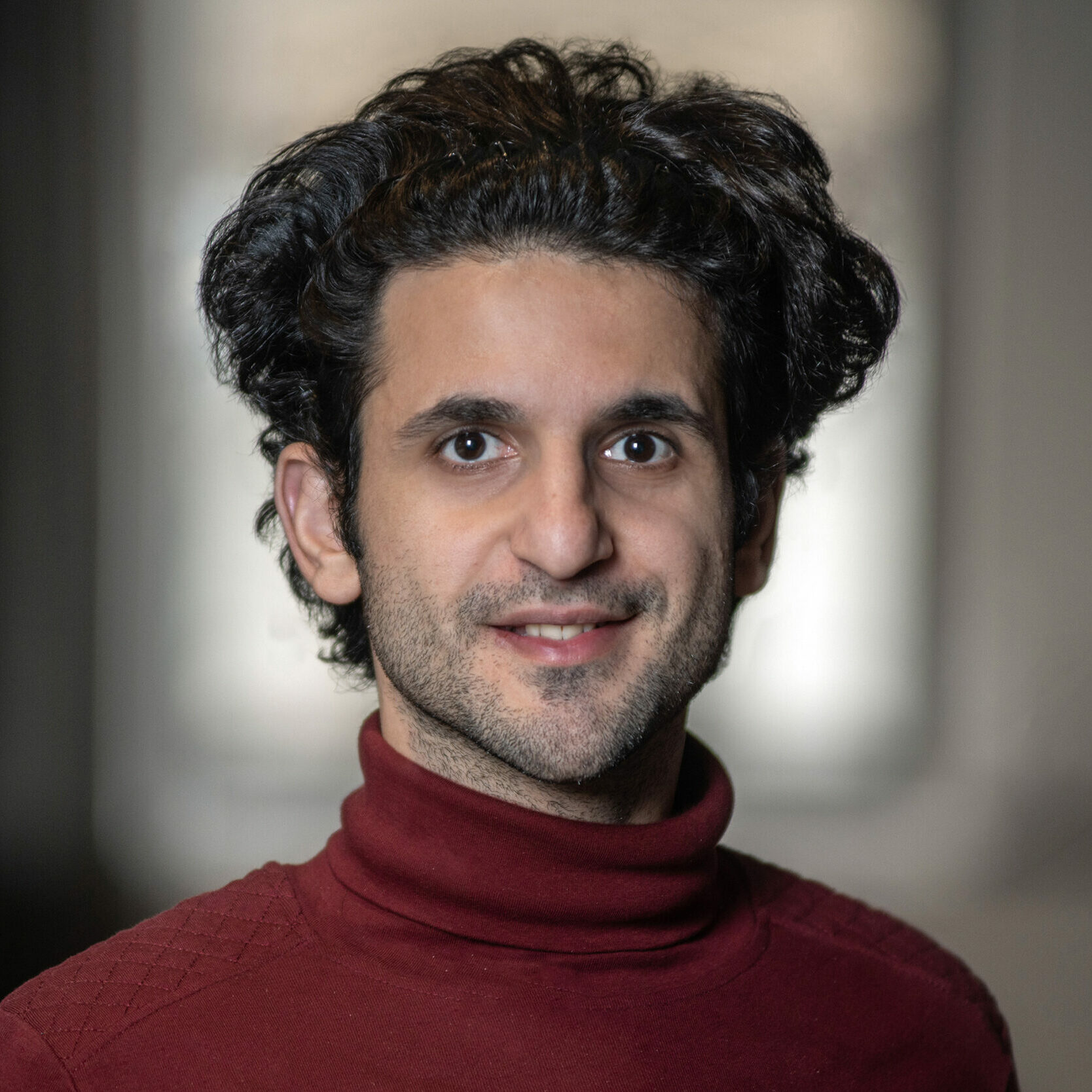
Amin Nejatbakhsh, PhD
2024 Leon Levy Scholar in Neuroscience
Flatiron Institute
Sub-disciplinary Category
Computational Neuroscience
Previous Positions
- BSc, Sharif University of Technology
- MA, Columbia University
- MPhil, Columbia University
- PhD, Columbia University (Advisor: Dr. Liam Paninski)
Bio
Dr. Amin Nejatbakhsh is a Flatiron Research Fellow in the Center for Computational Neuroscience and a Visiting Scholar at NYU. He completed his Ph.D. in the Center for Theoretical Neuroscience at Columbia University, primarily advised by Liam Paninski. Currently, he is a postdoctoral researcher in the lab of Dr. Alex Williams.
Research Summary
Statistical modeling of neural data to causally understand biological and artificial neural networks and the mechanisms therein.
Technical Overview
Neural dynamics during free behavior are far more complex than what researchers are observing in constrained task settings in a lab. To reduce the complexity, classical neuroscience experiments focused on simplified tasks, limiting our ability to understand neural systems when functioning in their natural environment. Experimental neuroscientists are now able to simultaneously record from large collections of neurons during rich, naturalistic behavior and sensory experiences, allowing them to gain invaluable insights, which were not possible with small-scale experimentation and extremely constrained task designs. However, with more neurons being recorded we need to collect more data to account for trial-by-trial variability, as the randomness or noise in neural signals plays a fundamental role in the representation and signaling capacity of neurons. Dr. Amin Nejatbakhsh proposes a set of statistical ideas that can be readily incorporated into current experimental pipelines to better understand the role of noise in neural representations and quantify individual differences in the population of animals during natural behavior. Dr. Nejatbakhsh plans to apply his methodologies to gain insight into visual and motor encoding across rodent, primate, and artificial models.